In the full-house customization industry, CAD drawings are a crucial way to present design projects. However, store designers’ CAD drawings cannot be directly recognized by machines in the the production and construction phase. Often, it requires manual conversion of the design language in the CAD drawings into three-dimensional models and further generates data for production line processing. This significantly impacts data accuracy, order splitting efficiency, and factory capacity.
To address this challenge, MANYCORE(Coohom) has been focusing on using AI for three-dimensional modeling in recent years. Recently, a paper titled “PlankAssembly: Robust 3D Reconstruction from Three Orthographic Views with Learnt Shape Programs”, co-authored with academic institutions like Sun Yat-sen University and the University of Electronic Science and Technology of China, was accepted by the International Conference on Computer Vision (ICCV). Along with ECCV and CVPR, ICCV is one of the top three international academic conferences in the field of computer vision.
Research project homepage: https://manycore-research.github.io/PlankAssembly
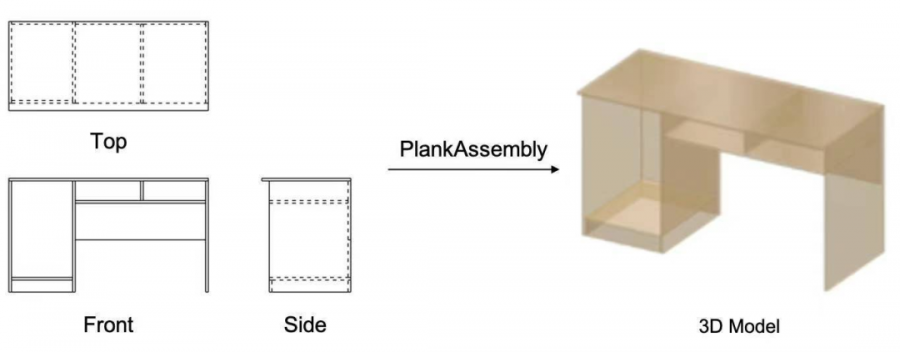
The paper explores a long-standing challenge in computer-aided design: reconstructing a 3D model from three views. Traditional algorithms solve this by establishing an explicit relationship between 2D drawings and 3D models. Experiments show that traditional algorithms are very sensitive to errors in drawings, limiting their application in actual products. Currently, some in the field have attempted to use geometric recognition to identify 2D information like length and width, but this only works for simple CAD model reconstructions.
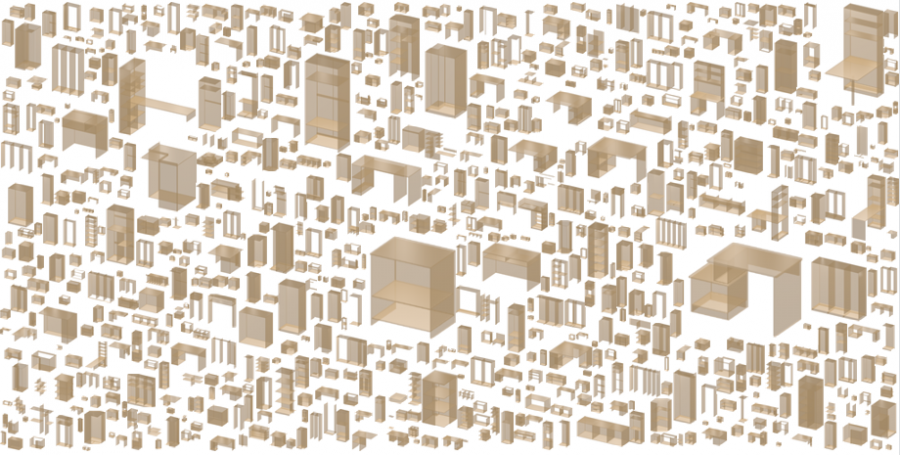
To address the sensitivity of algorithms to errors in drawings, MANYCORE has attempted to use AI deep learning algorithms based on data-driven methods. Specifically, this research employs a self-attention mechanism for implicit modeling. By training on a dataset containing over 26,000 parameterized CAD models, it can automatically convert two-dimensional line drawings from three orthographic views into three-dimensional CAD models. Compared to traditional algorithms, our proposed algorithm significantly improves noise robustness.
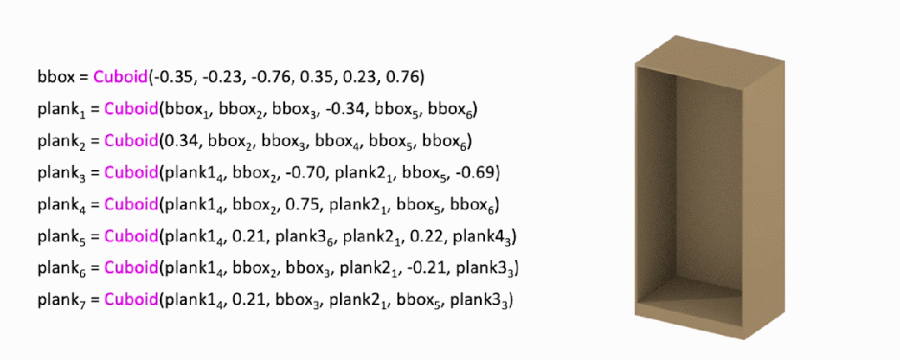
More importantly, while reconstructed objects are generally not editable, this research has reconstructed the interconnection between planks, allowing editable effects on reconstructed cabinets.
MANYCORE’s proposed algorithmic research provides the industry with a new approach to 3D modeling and has been supported by the Zhejiang Province Key Research and Development Program of 2022.
An AI industry professional expressed surprise at the potential impact of this research, describing it as a powerful tool for carpenters and 3D printers.
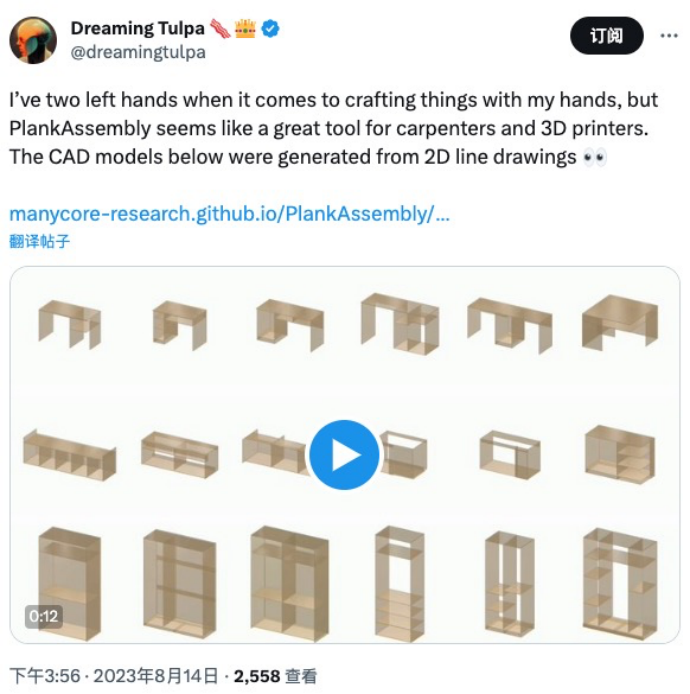
To quickly resolve the issue of CAD reconstruction for custom cabinets, MANYCORE will accelerate the industrial application of this research’s findings.
Contact support@coohom.com to learn more about our solutions.
Social Contact: